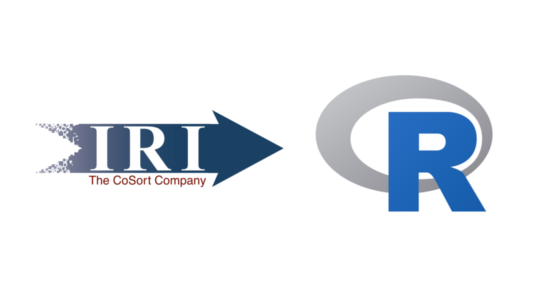
Easier Big Data Prep for R
Among analytic tools for statistical computation and graphics, R has shown an increase in popularity among data miners, and in the development of its open source language. However, from a performance standpoint, R holds all of its objects in virtual memory, which becomes an issue when attempting to work with very large data sets.
There are many ways to handle big data while still using R for the statistical analysis. Hadoop distillations come to mind, but they do not come cheaply or easily. Another obvious solution might be to add more memory to the PC where R runs (mine had 6GB). I tested the limits of how many rows R could handle at one time.
My R Limit
On my computer, about 3 million rows, or approximately 112MB, was all R could process at once. This amount of data is by no means part of the scope of “big data,” which is closer to the 100-million-row range. A little math and that means I’d need 200GB of RAM. That is still not feasible for PC users at this time.
Another option is to break the data down into smaller chunks that R can handle, process these chunks individually, and then summarize everything at the end. Let’s say you want to prepare 30 million rows worth of source data. With the aforementioned limitations of my PC, I had to break the data down into 10 chunks of 3 million rows each before R could process it.
With one simple line of code in R that handles “garbage collection,” you can remove the processed information from memory to make room for the next set. But to process these chunks before garbage collection, the R code needs to be in multiple files, all processed by one final summary code file. So if 30 million rows needs 11 R scripts, then 300 million rows would require an impractical 101 scripts.
A practical and time-saving solution is to use third-party software designed for pre-processing big data, so as to give R more manageable chunks to analyze. IRI CoSort is a data manipulation and management package that rapidly prepares, or franchises, raw data sources for BI and analytics using the existing Windows or Unix file system.
Comparing Options
Let’s use the same example as above, with 30 million total rows of data. Say you have one file with a list of store numbers, manager’s names, and state abbreviations. Then you have 30 million rows of data in another file(s) containing transaction information: account number, store number, item number, price, etc. We need to analyze sales revenue totals using the price of each item sold, grouped by each state (in Brazil) in which the item sold.
For R to process this information, the transaction data would have to be in 10 files of 3 million rows each to avoid memory overload. Each of these files requires its own R script to join with the store info file, then sort and sum the price based on state, with the totals saved to a results file You would then need an 11th R script to run the first 10 transform jobs, read the outputs of those, and create a final total based on state. Let’s assume this was your only option, and you performed all these steps. How long would it take?
Not counting the time it takes to write 11 R scripts, the run time for 30 million rows of data was 510.38 seconds, or 8 minutes and 16.51 seconds. The R scripts, shown via WalWare’s StatET for R — since updated to Eclipse StatET — in the center editing window of the IRI Workbench GUI below, look like this:
Here are the 10 data chunks I had to maintain:
before reaching the final summary result:
To obtain the same sort-sum process results with IRI CoSort, however, I needed just one CoSort ‘Sort Control Language’ (SortCL) program to sort and join two 15M-row files over their common key and sum them by state. SortCL supports any number (and size) of data sources and formats, and produces any number and type of targets simultaneously. See this functional summary diagram.
The SortCL job producing the same summary output as R (and in the same GUI) is shown here:
This way only took 272.95 seconds, or 4 minutes 32.95 seconds, which was 45% faster than R:
Plus, only having to write and manage one SortCL script, as opposed to 11 R scripts, saved even more time.
Conclusion
Either way, the raw data was distilled into the same subset R could quickly analyze and feed to a visualization tool like ggplot or qplot:
However, beyond the time-to-visualization advantage CoSort afforded me through simpler and speedier data preparation for R, I had centralized data that I could re-use, mask, and quality control in CoSort SortCL and compatible IRI software jobs.
This approach also avoids data being out-of-sync between R sessions that call for the same data at different times. Moreover, the data access and metadata management features in the IRI Workbench let me take and share control of the data life cycle, especially the remappings I wanted to do for R’s sake.
1 COMMENT
Good Article. The present day personal computing machines have not yet reached the state of satisfying the memory requirements of bigdata. One of the reasons why systems like hadoop are increasingly getting popular among new programmers. However, the alternate workflow indicated here about the workbench and external sorting looks interesting. Not sure how this works in production grade environment though. Anyway, thanks for sharing.
– Gopalakrishna Palem
M2M Telematics & Big Data Strategy Consultant
http://gk.palem.in/