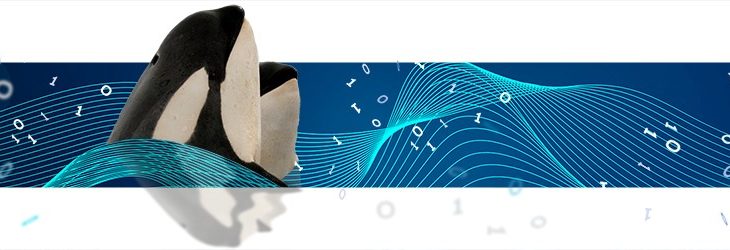
IRI Data Governance
In the previous article in this series, we discussed the importance of improving and maintaining the quality of your data. Along the same lines, it is also very important to make sure your data is well-governed. This is usually accomplished, as you might expect, by a data governance solution.
But what does that mean, exactly? The details vary from vendor to vendor, but most of the time it refers to a platform that can do all, or at least most, of the following: provide centralized data and metadata management; help you ensure data privacy and thus regulatory compliance, for instance via role-based access controls; manage and enforce enterprise-level policies (“data of this type must be protected in this way”), and provide self-service data access and/or automated data delivery. Other common capabilities include sensitive data discovery, data masking, data lineage, and data quality.
Essentially, data governance offers a way to look at and manage – and indeed, govern – your data landscape in a holistic fashion. The term “data stewardship” is sometimes also thrown around to refer to the care and management of specific pieces or collections of data assets, essentially working towards the same ends but operating at a somewhat lower level. You could even think of data governance as a way to enable data stewardship at an enterprise scale.
The primary tool of data governance in recent years has been the data catalog. Catalogs are essentially enterprise data and metadata management systems that provide a centralized, easy-to-use point of access for all of your data and metadata. They, therefore, provide a good lens through which the data governance methods described above can be used. They are also frequently very good at enabling collaboration and tracking the relationships between data assets, which can be important for, say, data privacy. Moreover, it is increasingly in vogue to tie governance assets (business terms, regulations, policies, and so on) to lower-level data and metadata assets, in order to imbue the latter with an appropriate business context and demonstrate its business value. Data catalogs are an excellent medium for doing this.
The benefits of data governance are both broad and substantial. You need a plan for regulatory compliance if you want to avoid hefty fines and reputational damage, and hence you need data privacy. But data privacy needs to be applied holistically to actually achieve (and maintain) regulatory compliance, which naturally leads to data governance, policy management, and so on. On the other side of things, your users need to be able to access data that is relevant to them efficiently and reliably, and self-service follows from that. But you can’t allow any user to access any piece of data regardless of the role of the former and the sensitivity of the latter, so you need role-based access controls and other such things in place, again leading back to data governance. In this sense, at least, data governance is a way of brokering between the needs of the individual user and the needs of the business as a whole.
Figure 1 – Privacy regulation around the world
IRI provides data governance via IRI Voracity, its “total data management” platform that runs the gamut in terms of centralized, consolidated enterprise data and metadata management capabilities. To start with, we’ve already highlighted some of the relevant capabilities it offers in previous blog posts (including data quality) which we won’t go over again here. More than that, it delivers data discovery and profiling, data masking, role-based access controls, data lineage (as well as through integration with Erwin), data reconciliation, risk scoring (say, for reidentification of anonymized data), and more as part of its data governance capabilities.
For discovery, in particular, Voracity offers an impressive range of methods for finding sensitive data, including pattern matching, named entity recognition (which leverages semi-supervised machine learning), column name matching, fuzzy and exact dictionary matching, path searching, facial recognition matching, font matching, character recognition, and coordinate matching. Any number of these methods can be used together for additional accuracy. In addition, for data reconciliation, you can reconcile disparate values while also amending them to comply with your formatting, privacy, and business rules.
All of this makes IRI Voracity a very capable back-end for data governance. Its most substantial shortcoming is that it doesn’t expose this back-end in a particularly user-friendly way, but IRI is aware of and working to address this. For instance, it is currently developing more centralized role-based access control capabilities, as well as an enhanced – and more API-driven – data classification infrastructure in IRI Workbench. Don’t misinterpret this: we’ve established earlier in this blog series that Voracity is easy to work with from a developer perspective, and that remains true, but from the perspective of a data governor used to a web front-end, it may be less accessible than desired, at least for the time being. On the other hand, put Voracity together with a front-end specifically designed for end users (Erwin or DataSwitch for example – both of which Voracity already integrates with) and you’ve got the best of both worlds.