Data Loss Prevention and Sensitive Data Protection
Data loss prevention (DLP) activities start with the profiling of data at risk, be it in motion or at rest. Next is the protection of that data with the proper application of security functions and protocols. Together these activities constitute a powerful form of content-aware DLP.
Leading DLP solutions offer scanning, filtering, highlighting, and monitoring solutions (to enforce protections) for data at risk. The granular data classification, discovery and de-identification technology in the IRI data masking tools:
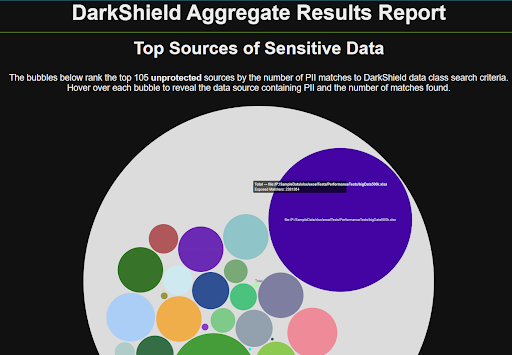
- FieldShield for finding and masking classified data in RDBs and flat files
- CellShield for doing the same, but within and across Excel® spreadsheets
- DarkShield for the same sources above, plus semi- and unstructured sources
- Voracity for all the above, plus cleansing, integrating, and wrangling all that data
can work alone or in tandem with other data classification and scanning tools to allow authorized users to: profile (classify and search), protect (mask or delete), and prove (risk score and audit) they acted to prevent -- or at least nullify -- the loss of sensitive data. Learn more in the tabs below.
Profile (Discover)
Classify, profile, and scan sources of sensitive data through location and content-based searches of multiple sources simultaneously. Identify, isolate, diagram and report on data at multiple table, files, and other sources at once. IRI DarkShield search and masking logs and the dashboard charts you can produce from them produce heat maps of ranked data risk like these:
When data is in flat files or databases, IRI FieldShield can also protect it from misuse. Built in data format (composite) template and range capabilities provide for content-aware identification and validation of columnar values. DarkShield uses the same data class definitions to locate and report on those values too, as well as data in semi-structured and unstructured data sources on-premise and in the cloud.
Protect (Mask)
Choose and apply built-in or custom data masking functions for sensitive fields. Choose which function to apply based on your need for:
- Security - how strong the encryption or other algorithm needs to be
- Speed - which functions conceal data (and/or reveal) faster
- Reversibility - whether you need to re-identify the data later
- Appearance - if the ciphertext needs to retain the original format
Apply these functions ad hoc or en masse (consistently across sources) using rules. For example, use pattern-matching expressions to automatically apply a format-preserving encryption key to certain tables, while using another key on others. This consistency in data masking function application preserves referential integrity.
Direct the output to the same source or new target. Assert both data- and role-based access controls that persist, wherever the data may later exist. This goes well beyond what other encryption-only or DLP-centric solution providers offer.
Prove (Audit)
Verify that you protected or de-identified the data at risk with statistical output and an audit trail. Job stats show column names, number of rows input/protected/output, and more.
The job specification script itself is self-documenting and easy to review in a text editor or in the GUI. It is also automatically integrated into a query-ready XML audit file. That log file also contains system information; e.g. who ran the job, where, and when.
Together with the sources and targets they identify, these records help validate the work you did to comply with data privacy laws.